CITI BIKE STRATEGY: MANHATTAN
- Sanjit_3282
- Nov 1, 2018
- 2 min read
Citi Bike is a privately-owned public sharing system and implemented in New York City in 2013. Citi Bike NYC needs to solve a docking issue in Manhattan due to the limited slots in each station, especially in the morning rush hour, as sometimes the stations are empty with no available bikes, or too full with no parking slots. This research solves the problem by cleaning and digging into the Citi Bike trip data.
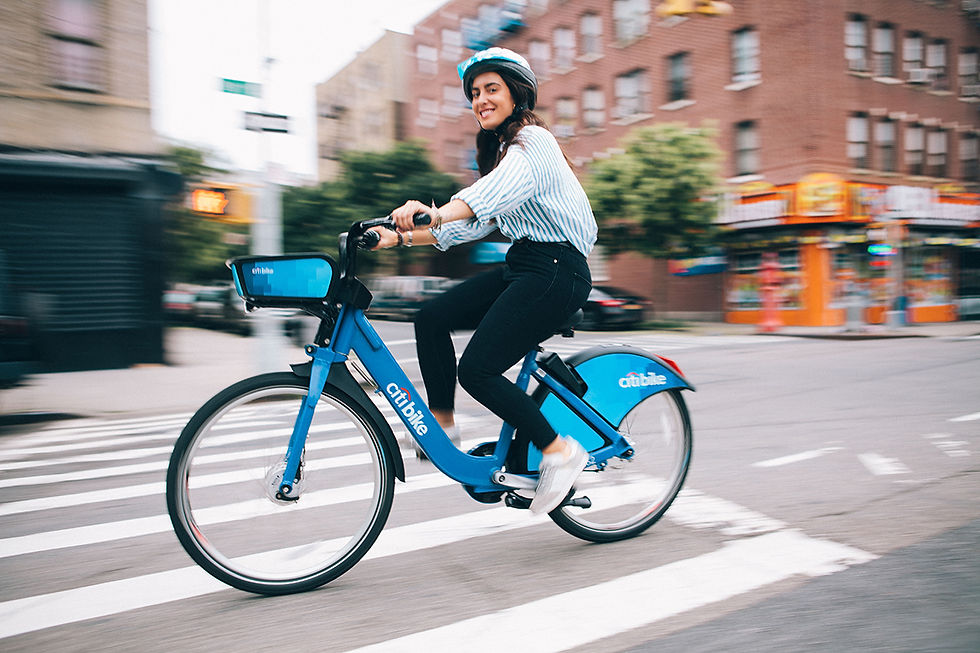
To analyze the Citi bike usage pattern across Manhattan and the influence of weather and taxi usage on it, our data included three parts, the Citi Bike trip data (550K+ trips) from their official website Citi Bike NYC, the weather data from Weather Underground, and the cab usage data (Yellow and Green Taxi) from Taxi & Limousine Commission of NYC (2.75Million+ trips) for the month of May and June 2017.
We merged the Citi bike data with taxi data based on the zones used for taxi trip’s starting and end point. The zones for Citi bike were created by grouping bike stations based on the longitude and latitude of taxi zones. Finally, weather data was merged based on the date info. The final step in the data preparation before merging in weather data was clustering start stations for easier modeling. We decided to go with 10 clusters because of the evenness of distribution of stations in each cluster, and the fact that 10 clusters captured the different neighborhoods of Manhattan well.
We found patterns of traffic loads seen by clusters using Bayesian Network and Apriori association. We first built a Bayes Net to find probabilistic and causal relationships in the data. The target variable in this model was the binned number of trips with all other variables as possible predictors. With a better understanding of the relationships between the variables, we moved forward to form strong association rules using an Apriori model.

Using Apriori model, we ended up with 10 strong rules for our analysis. We found that day of the week is the most important factor in determining the number of trips, whereas weather is less significant. Upper Manhattan sees the least traffic, whereas Midtown sees highest traffic even on rainy days and stations around Central park see high traffic during the weekends.
Based on our research, we have two suggestions. First, on weekdays with rains, move bikes to midtown and away from outer edges of Manhattan and second, on weekends with no rain, move bikes closer to Central Park. Below is the video of presentation for your reference. This was a team project and my team members were Evan DeCastros, Yicong Hu and YuanYuan Pei.
Comments